Merged-Logic Memory Fabric
Theme 4 (Merged Logic-Memory Fabric), is a holistic and ambitious cross-layer design, optimization and implementation effort to create a scalable, high-performance and energy-efficient microsystem that incorporates critical nanotechnology components from Themes 1-3.
The key elements of the proposed computation platform that can provide 1,000× in computing performance (energy-latency product) for future memory-intensive computing workloads such as real-time image/video recognition, on-line learning, big-data analytics, secure computing are: massive on-chip memory co-located with highly energy-efficient computation, enabled by “monolithic 3D integration” using ultra-dense and fine-grained massive connectivity and complemented by advanced packaging technologies that provide low system cost.
The materials and device technologies developed in all four Themes will support not only conventional computing architectures but also architectures that are currently under intense research, ones that embrace sparsity, stochasticity, and nanodevice variability, including those that are associative, machine/deep learning-driven, and homomorphic encrypted. While deep neural networks have already enabled large-scale machine learning applications on the cloud, today’s cognitive hardware suffer from long training times, large training datasets, lack of flexibility and dynamic adaptation, and real-time autonomous decision-making.
Leverage innovations in novel device technologies to create merged logic-memory fabrics to accelerate cognitive and secure computing workloads.
Theme 4 will pursue and harness breakthroughs in novel devices (such as multi-state analog memories, cross-point memories, vertical 3D memories) in conjunction with breakthroughs in architectures and computational models (such as processing-in-memory, hyper-dimensional computing, approximate computing) to demonstrate cognitive hardware that overcomes current limitations through dynamic adaptation and parallel on-line learning. Novel integration technologies will enable solving large real world at-scale problems in the capacity limited regime, while enabling ubiquitous intelligence in the energy constrained setting.
We expect Theme 4 to have extensive technical coupling to other five centers within the JUMP community, to jointly conduct application and algorithm level evaluation and optimization of the next generation secure and cognitive integrated microsystems.
Affiliated Investigators
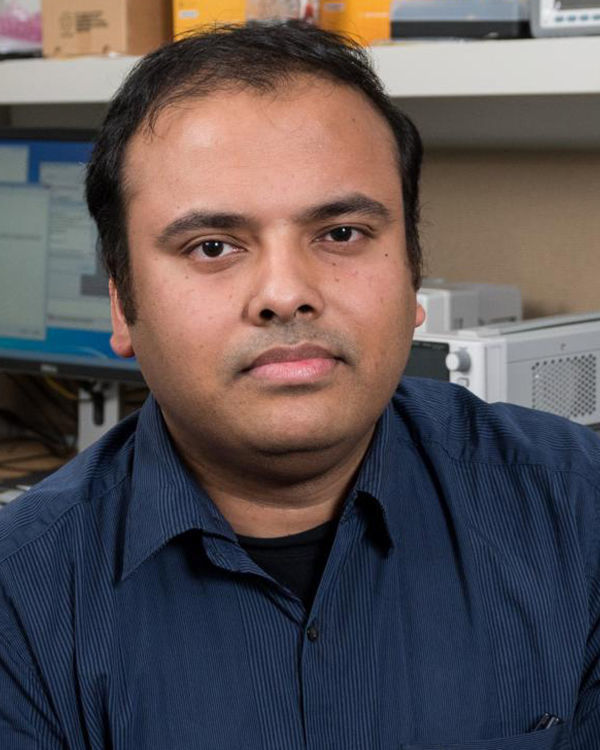
Arijit Raychowdhury
Electrical and Computer Engineering Georgia Tech